
Bits With Brains
Curated AI News for Decision-Makers
What Every Senior Decision-Maker Needs to Understand About AI and its Impact
AI Plays God: Rewriting the Book on Drug Discovery
11/3/24
Editorial team at Bits with Brains
The biotechnology sector is experiencing a seismic shift as artificial intelligence (AI) takes root
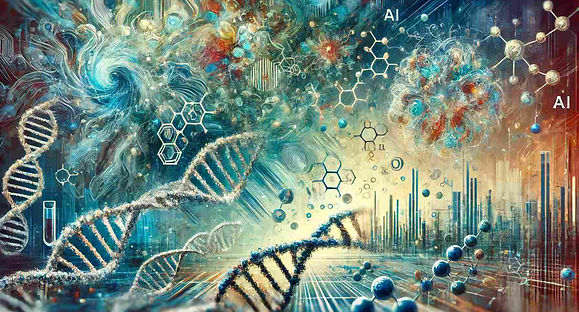
Key Takeaways
AI is revolutionizing drug discovery by overcoming traditional challenges in target identification, lead discovery, and drug testing.
Machine learning and deep learning algorithms, particularly Convolutional Neural Networks (CNNs) and Generative Adversarial Networks (GANs), are playing crucial roles in various stages of drug discovery.
AI-powered virtual screening and molecular design are significantly accelerating the process of identifying promising drug candidates.
The use of AI in drug discovery has the potential to cut development timelines in half and reduce costs by up to 30%, according to a McKinsey & Company study.
AI tools like TxGNN are being developed to address rare diseases and conditions with no existing treatments, offering free access to clinician-scientists to advance therapeutic research.
AI Shakes Up Biotech: Ginkgo's Bold Move and Harvard's Game-Changing Tool
The biotechnology sector is experiencing a seismic shift as artificial intelligence (AI) takes root. Two recent developments – Ginkgo Bioworks' ambitious AI initiative and Harvard's innovative drug repurposing tool – are at the forefront. Their advancements promise to reshape how we approach drug discovery, synthetic biology, and the treatment of rare diseases.
Here’s a look into these groundbreaking developments and explore their potential impact on the future of medicine.
Ginkgo Bioworks: The "OpenAI of Biotech"?
Ginkgo Bioworks is a synthetic biology company with some impressive AI accomplishments:
They've launched a protein-focused large language model (LLM) and an API for synthetic biology AI systems. This LLM is specifically designed to assist in protein building, a crucial aspect of synthetic biology and drug development. The API allows researchers and developers to access not only this model, but also other AI systems related to synthetic biology, creating a comprehensive toolkit for biotech innovation.
The company is positioning itself as the "OpenAI or Anthropic" of biotech, aiming to create a developer marketplace. This ambitious goal reflects Ginkgo's vision of becoming a central hub for AI-driven biotechnology tools and services. By emulating the success of major AI companies in the tech sector, Ginkgo hopes to foster a thriving ecosystem of biotech developers and researchers.
This marks a significant shift in Ginkgo's business model, moving from problem-solving to tool-providing. Previously, Ginkgo focused on directly addressing biological challenges for clients. Now, they're pivoting to provide the tools and infrastructure for others to solve these problems, potentially scaling their impact across the entire industry.
The initiative builds on Ginkgo's existing partnership with Google Cloud, announced in 2023. This collaboration likely provides the computational power and expertise needed to develop and deploy large-scale AI models for biotechnology applications.
Ginkgo's strategy could democratize access to powerful biotech tools, potentially accelerating innovation across the industry. By providing a platform for developers and researchers to access advanced AI models and tools, Ginkgo could lower the barriers to entry for biotech innovation. This could lead to a more diverse range of players in the field, from small startups to academic researchers, all contributing to advancements in synthetic biology and drug discovery.
However, the company needs this pivot to pay off after facing financial challenges. Ginkgo has experienced mass layoffs and stock price declines, making the success of this new direction crucial for the company's future. The shift from a service-based model to a platform-based one is a high-stakes move that could either solidify Ginkgo's position as a leader in the biotech industry or further strain its resources if it fails to gain traction.
Harvard's TxGNN: AI-Powered Hope for Rare Diseases
Meanwhile, Harvard Medical School researchers have developed TxGNN, an AI model for drug repurposing that's generating excitement in the medical community:
The tool identifies existing drugs that could treat rare diseases, addressing a major unmet medical need. With approximately 300 million people worldwide affected by rare diseases, many of which lack effective treatments, TxGNN could be a game-changer in improving patient outcomes.
TxGNN outperforms other models in identifying drug candidates and predicting contraindications. Specifically, it's claimed to be 50% better at identifying potential drug candidates and 35% better at predicting contraindications compared to leading repurposing models. This improved accuracy could significantly reduce the time and resources spent on drug trials that are likely to fail.
It's been trained on extensive public data and validated using real patient records. The model draws from public data repositories including DNA information and clinical notes, providing a broad base of knowledge. Its validation on 1.3 million deidentified patient records from Mount Sinai Hospital in New York lends credibility to its predictions and demonstrates its potential real-world applicability.
TxGNN includes an explainer component to elucidate the reasoning behind its predictions. This transparency is crucial for building trust among clinicians and researchers who may be skeptical of AI-driven recommendations. It also aids in understanding the biological mechanisms behind the drug-disease interactions predicted by the model.
TxGNN is freely available to scientists, potentially fast-tracking discoveries for diseases that often lack research funding. By making this powerful tool accessible at no cost, Harvard is enabling researchers worldwide to explore new treatment possibilities for rare diseases. This open approach could lead to collaborative efforts and breakthroughs that might not have been possible with traditional, siloed research methods.
The Bigger Picture: AI's Growing Role in Biotech
These developments highlight some key trends that are reshaping the biotechnology landscape:
AI-driven innovation is becoming central to biotechnology and drug discovery. The integration of AI into these fields is not just an add-on but a fundamental shift in how research and development are conducted. AI's ability to process vast amounts of data and identify patterns is proving invaluable in areas like protein folding, drug-target interaction prediction, and genetic analysis.
High-quality, large-scale biological data is crucial for advancing AI applications in this field. Both Ginkgo's initiative and Harvard's TxGNN underscore the importance of comprehensive, reliable data sets in training effective AI models. This emphasis on data quality and quantity is driving efforts to improve biological data collection, standardization, and sharing across the scientific community.
There's a push to democratize access to powerful AI tools in biotech. By making advanced AI models and tools more accessible, companies and institutions are fostering a more inclusive innovation ecosystem. This democratization could lead to a diversity of approaches and ideas, potentially accelerating the pace of discovery and development in biotechnology.
These tools could significantly reduce the time and cost of drug discovery and development. Traditional drug development is a lengthy and expensive process, often taking over a decade and billions of dollars to bring a new drug to market. AI-driven approaches, particularly in areas like drug repurposing, have the potential to dramatically shorten this timeline and reduce costs, making drug development more efficient and potentially more accessible to a wider range of conditions and patient populations.
What’s Next?
The integration of AI in biotechnology holds immense promise, with far-reaching implications for healthcare and scientific research:
Faster drug discovery: Repurposing existing drugs could bring treatments to patients more quickly, especially for rare diseases. AI models like TxGNN can rapidly identify potential drug candidates from vast libraries of existing compounds, potentially shaving years off the drug discovery process. This is particularly crucial for rare diseases, where traditional drug development approaches often fall short due to limited market incentives.
More efficient research: By making powerful tools accessible, we could see a surge in biotech innovation from a wider range of researchers and companies. Smaller labs and startups that previously lacked the resources for large-scale drug discovery efforts could now leverage AI tools to compete with larger pharmaceutical companies. This democratization of research capabilities could lead to a more diverse and innovative biotech ecosystem.
Potential cost savings: AI-driven approaches could reduce the astronomical costs associated with traditional drug development. By improving the accuracy of early-stage drug candidate selection and streamlining the development process, AI could help allocate resources more efficiently. This could potentially lower the overall cost of bringing new treatments to market, which could translate to more affordable medications for patients.
Personalized medicine advancements: The ability of AI to analyze vast amounts of genetic and clinical data could accelerate progress in personalized medicine. Tools like TxGNN, which can draw parallels between different diseases based on genomic underpinnings, could help tailor treatments to individual patients' genetic profiles, improving efficacy and reducing side effects.
However, it's important to temper excitement with caution. The real-world performance of these tools remains to be seen, and their adoption will likely face regulatory and ethical hurdles. Issues such as data privacy, the potential for bias in AI models, and the need for clinical validation of AI-generated predictions will need to be carefully addressed as these technologies move from the lab to clinical applications.
As AI continues to reshape biochemistry and pharmacology, we're entering an era of unprecedented potential for medical breakthroughs. The convergence of big data, machine learning, and biological sciences is opening new frontiers in our understanding of life and our ability to intervene in disease processes.
The coming years will likely see an acceleration of AI integration in biotechnology, with potential impacts ranging from more efficient drug discovery pipelines to novel insights into fundamental biological processes. As these technologies mature, we may witness a transformation in how we approach healthcare, moving towards more predictive, preventative, and personalized models of medicine.
FAQ
Q: How does AI help in drug discovery?
A: AI helps in drug discovery by analyzing large datasets of patient health records, protein structures, and existing drugs to identify potential drug targets and molecules. It also assists in virtual screening, lead optimization, and predicting drug-target interactions, significantly speeding up the process and reducing costs.
Q: How will generative AI disrupt data science in drug discovery?
A: Generative AI, particularly Generative Adversarial Networks (GANs), is disrupting drug discovery by creating novel drug-like molecules. This approach allows for the rapid generation and evaluation of potential drug candidates, potentially leading to more innovative and effective treatments.
Q: What are the main challenges in traditional drug discovery that AI addresses?
A: AI addresses several key challenges in traditional drug discovery, including:
Time-consuming target identification
Resource-intensive lead discovery
Lengthy and expensive drug testing processes
Limited ability to repurpose existing drugs for new treatments
Q: How accurate are AI models in drug discovery?
A: While accuracy can vary depending on the specific application, some studies have shown promising results. For example, a study published in Nature Biotechnology in 2020 demonstrated that AI models exhibited improved accuracy in identifying drug targets for Alzheimer's disease compared to conventional approaches.
Q: Are there any AI tools available for researchers to use in drug discovery?
A: Yes, tools like TxGNN, developed by Harvard's Zitnik lab, are being made available for free to encourage clinician-scientists to advance the search for new therapies, particularly for rare diseases and conditions with limited treatment options.
Sources:
[1] https://www.emergingtechbrew.com/stories/2024/10/04/ginkgo-bioworks-api-synthetic-biology-ai-models
[3] https://aadcnews.com/news/ai-model-identifies-existing-drugs-repurpose-rare-diseases/
[6] https://www.emergingtechbrew.com/stories/2024/10/16/harvard-ai-tool-drugs-repurposing-rare-diseases
Sources